KR Webzine Vol.129
- Dec. 2021
- Nov. 2021
- Oct. 2021
- Sep. 2021
- Aug. 2021
- Jul. 2021
- Jun. 2021
- May. 2021
- Apr. 2021
- Mar. 2021
- Feb. 2021
- Jan. 2021
- Dec. 2020
- Nov. 2020
- Oct. 2020
- Sep. 2020
- Aug. 2020
- Jul. 2020
- Jun. 2020
- May. 2020
- Apr. 2020
- Mar. 2020
- Feb. 2020
- Jan. 2020
- Dec. 2019
- Nov. 2019
- Oct. 2019
- Sep. 2019
- Aug. 2019
- Jul. 2019
- Jun. 2019
- May. 2019
- Apr. 2019
- Mar. 2019
- Feb. 2019
- Jan. 2019
- Dec. 2018
- Nov. 2018
- Oct. 2018
- Sep. 2018
- Aug. 2018
- Jul. 2018
- Jun. 2018
- May. 2018
- Apr. 2018
- Mar. 2018
- Feb. 2018
- Jan. 2018
- Dec. 2017
- Nov. 2017
- Oct. 2017
- Sep. 2017
- Aug. 2017
- Jul. 2017
- Jun. 2017
- May. 2017
- Apr. 2017
- Mar. 2017
- Feb. 2017
- Jan. 2017
- Dec. 2016
- Nov. 2016
- Oct. 2016
- Sep. 2016
- Aug. 2016
- Jul. 2016
- Jun. 2016
- May. 2016
- Apr. 2016
- Mar. 2016
- Feb. 2016
- Jan. 2016
- Dec. 2015
- Nov. 2015
- Oct. 2015
- Sep. 2015
- Aug. 2015
- Jul. 2015
- Jun. 2015
- May. 2015
- Apr. 2015
- Mar. 2015
- Feb. 2015
- Jan. 2015
- Dec. 2014
- Nov. 2014
- Oct. 2014
- Sep. 2014
- Aug. 2014
- Jul. 2014
- Jun. 2014
- May. 2014
- Apr. 2014
- Mar. 2014
- Feb. 2014
- Jan. 2014
- Dec. 2013
- Nov. 2013
- Oct. 2013
- Sep. 2013
- Aug. 2013
- Jul. 2013
- Jun. 2013
- May. 2013
- Apr. 2013
- Mar. 2013
- Jan. 2013
- Dec. 2012
- Nov. 2012
- Oct. 2012
- Sep. 2012
- Aug. 2012
- Jul. 2012
- Jun. 2012
- May. 2012
- Apr. 2012
- Mar. 2012
- Feb. 2012
- Jan. 2012
- Dec. 2011
- Nov. 2011
- Oct. 2011
- Sep. 2011
- Aug. 2011
- Jul. 2011
- Jun. 2011
- May. 2011
- Apr. 2011
- Mar. 2011
- Feb. 2011
- Jan. 2011
- Dec. 2010
- Nov. 2010
- Oct. 2010
- Sep. 2010
- Aug. 2010
- Jul. 2010
- Jun. 2010
- May. 2010
- Apr. 2010
- Mar. 2010
- Feb. 2010
- Jan. 2010
- Dec. 2009
- Nov. 2009
- Oct. 2009
- Sep. 2009
- Aug. 2009
- Jul. 2009
- Jun. 2009
- May. 2009
- Apr. 2009
- Mar. 2009
- Feb. 2009
- Jan. 2009
- Dec. 2008
- Nov. 2008
- Oct. 2008
- Sep. 2008
- Aug. 2008
- Jul. 2008
- Jun. 2008
- May. 2008
- Apr. 2008
- Mar. 2008
- Feb. 2008
11
November 2018
1. The Establishment of the Big Data Analysis Environment
In the maritime industry, the most common big data is mostly data related to ship operations, which is not easy to obtain for big data analysis. In order to overcome this limitation, KR has established an environment that can analyze the operational performance of the actual voyages of ships using only public big data.
KR has undertaken considerable research to obtain and pre-process the dynamic big data, ready for analysis, using AIS (Automatic Identification System) data including position, speed, course, destination, ETA (Estimate Time of Arrival), draft, and ocean weather data including wind speed and wind direction, wave height and wave direction and current, for each latitude and longitude.
In addition, KR has gathered and stored in a database the static data of the principal dimensions, engine specification, build date, ship owner, and ship builder, and so on of each ship. The outline of KR’s Big Data Analysis Environment is as shown below in Fig. 1.
Fig. 1 Outline of KR’s Big Data Analysis Environment
2. Development of Big Data Analysis Applications
In KR, all of this big data has been collected, processed, stored in a big data cluster, and sorted according to interrelationships such as time, latitude, longitude, and IMO number. As a result, now table-shaped data including the voyage route and the corresponding ocean weather for each ship can be generated. We have developed various applications as shown in Fig. 2, so that the data processed at any time can be accessed on the web, at a glance by visualizing the past voyage route of the ship, the ocean weather situation at that time, and the changes of speed.
Fig. 2 Ship Operational Profile Analysis Visualization on the basis of big data
Furthermore, KR has implemented an application that can estimate average speed, the number of days of operation in rough seas, DFOC (Daily Fuel Oil Consumption) and the brake horsepower for each vessel. Based on this, we can observe the unnecessary fuel consumption incurred using "Rush to Wait" operations, by analyzing the DFOC and FOC / nm (Nautical Mile) and the average speed of the ship operating on the same route, as shown in Fig.3.
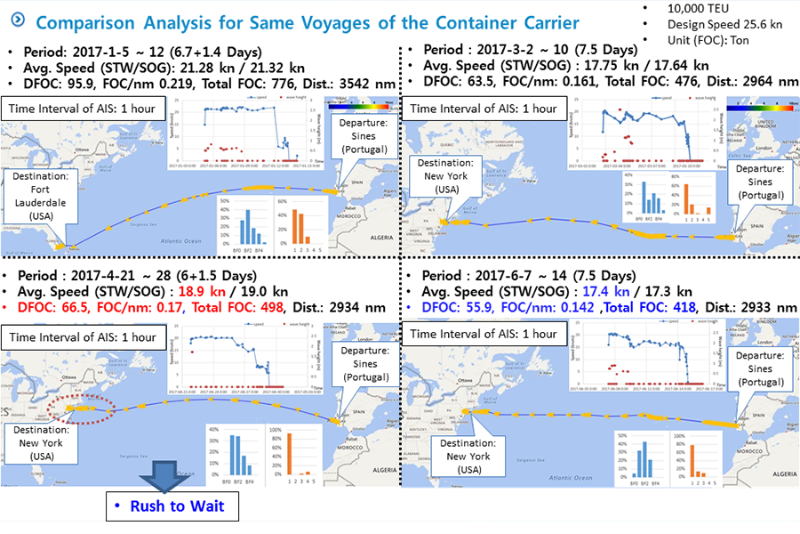
Fig. 3 Comparative analysis of the same route for a single ship
(showing the relationship between speed and fuel consumption)
The additional resistance resulting from the ocean weather environment (wind, wave, water temperature) is estimated using the ISO15016 method, and the total resistance is calculated according to the Holtrop-Mennen method to derive the speed-power curve for the actual operation of ship.
As a result of the analysis, KR developed a technique to compare and analyze the fuel efficiency of two different 13,000TEU class container ships (built in different shipyards and operated by different shipping companies) with the same design speed but very big differences in DFOC, as shown in Fig. 4.
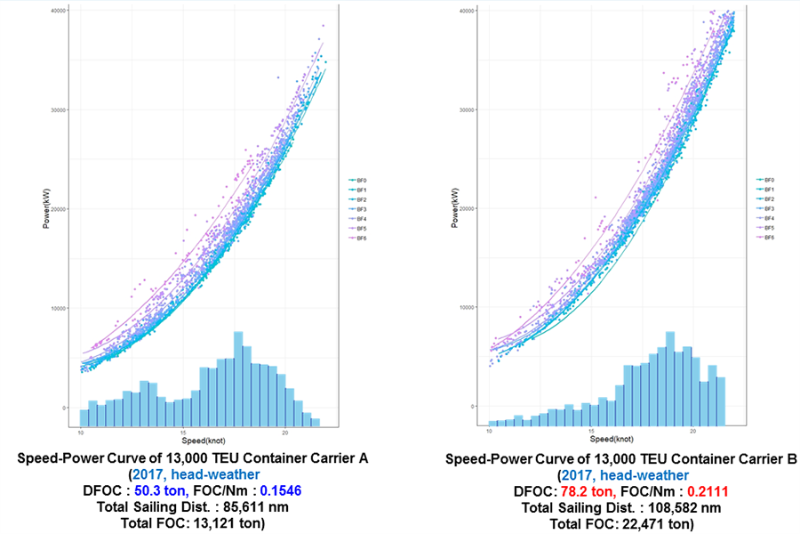
Fig. 4 Speed-Power Curve comparison between the voyages in 2017 of two different ships
with same design speed, and same cargo capacity
3. Big Data Analysis Application Service Development
KR has acquired, processed and analyzed 2017 data for about 4,000 ships of various types including container ships, LNG carriers, bulk carriers, and tankers, and 1,400 KR registered vessels, respectively to verify the availability of this big data analysis environment. In the future, we plan to enhance the application of big data by connecting and verifying with actual operational measurement data and supporting the customized vessel-specific or ship-type-specific analysis according to customer's request. Future work will be conducted using a newly developed predictive model applying deep learning technology from past operating data and ocean weather data, and we will carry out a study to predict the fuel oil consumption and the speed through water according to the route plan in a short time.